We are living in an era where machines can make decisions as complex and nuanced as humans, adapting to new situations with ease. This was reached thanks to neural network policies trained with large-scale reinforcement learning.
Before we dive into the deep end, let’s refresh our memory on reinforcement learning (RL). AI agent are now capable to explore a new playground (the environment). As it interacts with the swings and slides (states/observations), it makes choices (actions) and learns from the consequences which can be a successful somersault (reward) or the disappointment of a scraped knee (penalty). The AI agent goal? Learn and maximize the fun (cumulative rewards) over time.
A classic example of RL in action is an AI mastering the game of chess. Each move is an action, the board configuration is the state, and winning the game is the ultimate reward.
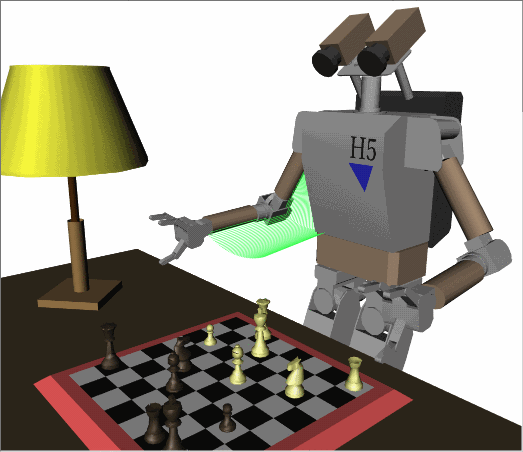
Now, let’s upgrade our playground explorer with a neural network brain.
A policy in AI is essentially a decision-making strategy. When we represent this policy as a neural network, we’re giving our AI agent the ability to learn incredibly complex relationships between what it observes (states) and what it should do (actions).
Let’s say our robot is learning to dance. A simple policy might be “move left foot, then right foot”. But a neural network policy can learn the intricate choreography of a tango, adapting to different music tempos and dance partners.
How this is possible? It’s all about practice through billions of practice sessions.
Let’s go through the process:
- Starting from Scratch: Our robot begins with random dance moves (random neural network parameters).
- Practice Makes Perfect: It starts dancing, making decisions based on its current skill level.
- Learn from Mistakes (and Successes): The outcomes of each dance session are used to tweak the neural network, gradually improving its performance.
But to reach great performances, it’s primordial to train the model using sophisticated techniques, such us:
- Policy Gradient Methods: Imagine a dance coach giving direct feedback. “More hip swing!” translates to adjusting the policy parameters for better performance. Algorithms like REINFORCE and Proximal Policy Optimization (PPO) fall into this category.
- Actor-Critic Methods: Picture a dance duo — the actor (policy) performs the moves, while the critic (value function) judges how well they’re done. This teamwork often leads to more graceful learning.
- Experience Replay: Think of this as watching recordings of past performances. By revisiting these stored experiences, the neural network can learn more efficiently, picking up on subtle details it might have missed the first time around.

Neural Network policy with RL approach in AI decision-making is giving computer and robots a super power:
- they can handle complex input, like the myriad of sensory data a self-driving car needs to process
- they learn end-to-end, and possibly discovering more creative ways humans may not even thought about.
The results are astonishing. This technology has led to AI that can beat world champions at Go (AlphaGo) and humanoids that can easily walk in San Francisco streets.
🎉 Keep learning and discovering AI news. I will be happy if you Follow me and Clap this post. Thanks 😸